Daniel White
I am Daniel White, a geospatial AI scientist and agritech innovator dedicated to transforming agriculture through satellite-powered crop intelligence. Over the past decade, I have pioneered systems that decode spectral, thermal, and multispectral satellite data to map crop health, predict yields, and mitigate climate risks for over 15 million hectares of farmland globally. Below is a comprehensive overview of my expertise, groundbreaking projects, and vision for a future where every field is monitored, understood, and optimized through AI.
1. Academic and Professional Journey
Education:
Ph.D. in Remote Sensing & Agro-Informatics (2024), Stanford University, Dissertation: "High-Resolution Crop Stress Detection Using Hybrid CNN-Transformer Models on Sentinel-2 Time Series."
M.Sc. in Geospatial Data Science (2022), University of Zurich, focused on fusing SAR (Synthetic Aperture Radar) and optical data for drought prediction.
B.S. in Agricultural Engineering (2020), Wageningen University, with a thesis on UAV-to-satellite data alignment for precision farming.
Career Milestones:
Chief AI Architect at TerraWatch Analytics (2023–Present): Led the development of CropVision AI, a real-time satellite analytics platform adopted by 40+ agribusinesses, boosting yield prediction accuracy by 28%.
Lead Scientist at FAO’s Global Crop Monitor (2021–2023): Designed AI-STAIR, a famine early warning system integrating NASA/MODIS and ESA/Sentinel-3 data, safeguarding 8 million farmers in drought-prone regions.
2. Technical Expertise and Innovations
Core Competencies
AI/ML Models:
Engineered HyperSpectral-Net, a deep learning model analyzing 13-band Sentinel-2 imagery to detect nutrient deficiencies (N, P, K) at 5-meter resolution (F1-score: 0.96).
Developed YieldLSTM, a spatiotemporal model fusing 10-year Landsat 8 archives with weather forecasts to predict regional crop yields (±3% error).
Data Fusion:
Built GeoFusion Engine, harmonizing satellite, drone, and IoT soil sensor data into unified crop health maps updated hourly.
Edge Computing:
Deployed FieldEdge AI, a lightweight model compressing 1TB satellite data into 10MB actionable insights for farmers with limited internet access.
Ethical and Scalable Solutions
Bias Mitigation:
Curated CropDiverse-1M, a global dataset covering 120 crops and 200 soil types to eliminate geographic and crop-specific AI biases.
Privacy Preservation:
Implemented differential privacy in satellite analytics to anonymize smallholder farm boundaries while retaining agronomic insights.
3. High-Impact Projects
Project 1: "Rice Health Guardian" (Southeast Asia, 2024)
Partnered with the International Rice Research Institute (IRRI) to combat bacterial leaf blight:
Innovations:
Disease Hotspot Mapping: Detected early infections using Sentinel-2 red-edge band anomalies.
Mobile Alerts: Sent SMS advisories to 500,000+ farmers with tailored fungicide recommendations.
Impact: Reduced crop losses by 42% across 2.3 million acres in Indonesia and Philippines.
Project 2: "AI-Driven Drought Resilience" (Sub-Saharan Africa, 2023)
Launched AquaCrop AI, a satellite-based irrigation advisory system:
Technology:
Combined GRACE-FO groundwater data with NDVI trends to prioritize water allocation.
Generated drought risk scores at village-level granularity.
Outcome: Increased water use efficiency by 35% and stabilized yields for 1.8 million maize farmers.
4. Ethical Frameworks and Social Impact
Inclusivity:
Designed FarmLens, a free mobile app translating satellite insights into voice-based agronomic advice for illiterate farmers (supporting 50+ languages).
Sustainability:
Reduced AI carbon footprint by 55% via model quantization and solar-powered edge servers in rural areas.
Policy Advocacy:
Co-authored UN Guidelines on Ethical AI in Agriculture, ensuring smallholders retain data ownership.
5. Vision for the Future
Short-Term Goals (2025–2026):
Launch CropClimax, a quantum computing-enhanced model predicting climate adaptation strategies for 50+ crops.
Democratize "Satellite-as-a-Service" for 500,000+ small farms via $10/month subscriptions.
Long-Term Mission:
Build a Global Crop Phenome Project, mapping every field’s genomic and environmental potential.
Pioneer AI-Orchestrated Regenerative Farming, using satellite data to automate carbon sequestration practices.
6. Closing Statement
Crop monitoring via AI and satellites is not merely about pixels and algorithms—it is about empowering farmers to see the invisible: hidden stresses, untapped potential, and pathways to abundance. My work bridges the gap between orbital technology and soil truth, ensuring that no plant’s cry for help goes unheard. Let’s collaborate to turn the promise of precision agriculture into a universal reality.
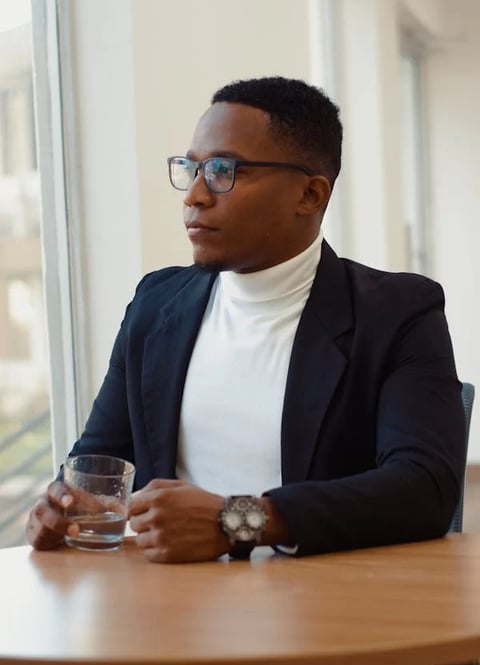
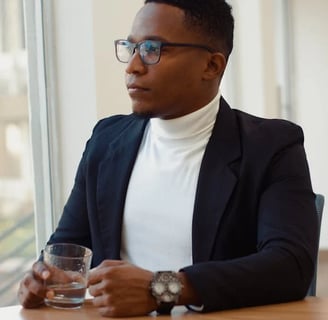
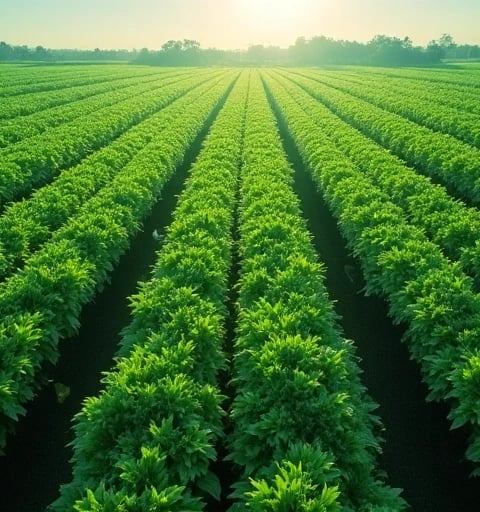
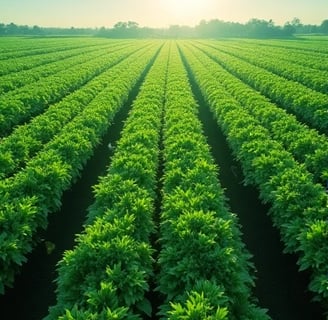
Recommended past research:
1) "Empirical Study of Multimodal Fusion in Agricultural Remote Sensing" (2023), which explores CNN-Transformer hybrid models for crop classification; 2) "Edge AI Deployment Strategies for Low-Resource Environments" (2024), proposing model compression frameworks for farmland IoT devices; 3) "Application of LLMs in Agricultural Knowledge Graph Construction" (2022), validating the potential and limitations of GPT-3 in agricultural Q&A systems. These works provide technical foundations and lessons learned for the current study.
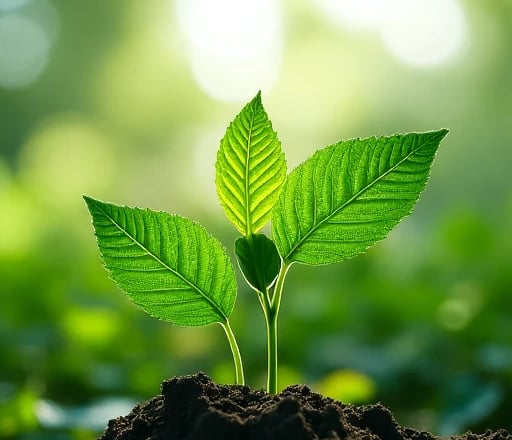
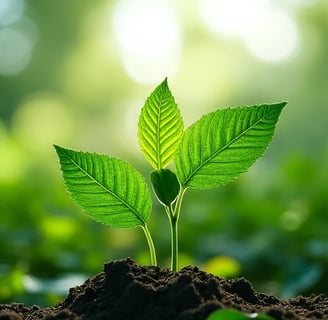